Artificial Intelligence Helps MITRE Save Lives During the Pandemic
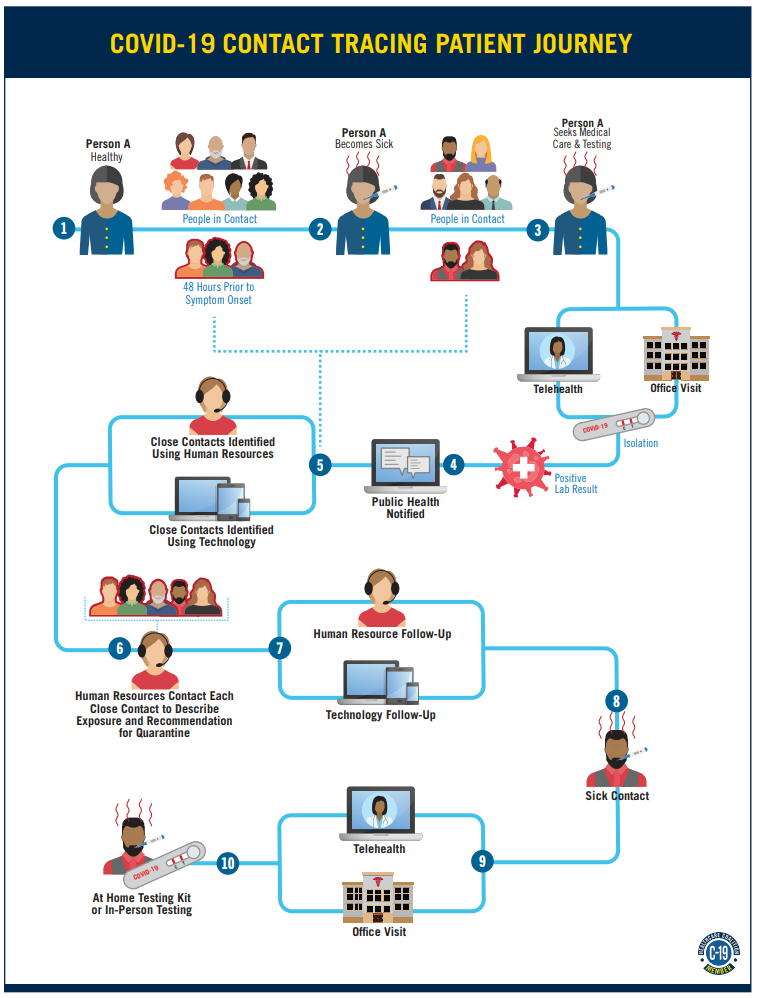
Credit: MITRE COVID-19 Healthcare Coalition
Author: Omkar Ratnaparkhi
This story is the second in a series about how MITRE tackles complex problems. Two stories on how MITRE’s student research programs develop researchers who want to work in the public interest will round out this series.
Modeling Enables Disciplined Problem Solving
A computer scientist looks at a refugee crisis to model a pandemic.
How does that connection work exactly and why? If it appears to resemble a scenario in which a farmer learns how to plant an apple orchard by examining the supply chain for manufacturing orange juice, well, you may actually be onto something. The novel coronavirus has presented unprecedented medical, social, and economic challenges, which means that the thoughtful people responding have no choice but to innovate. However, in 2020, innovation doesn’t mean going where no one has gone before. It means harvesting everything that has come before in a disciplined way to see what might bear fruit.
According to MITRE Technical Fellow Jay Crossler, “MITRE has three north stars for its healthcare coalition—data-driven clinical outcomes, supply chain, and social policies—and each of them has an AI component.” Using artificial intelligence and machine learning (AI/ML) tools to model personal protective equipment (PPE) needs and the effects of social policy proposals are just two of the areas on which MITRE’s modeling experts are focusing.
Modeling uses algorithms to simulate scenarios that help researchers answer questions. Are the models perfectly accurate? No, but perfection is not the point. What models offer is a common baseline of understanding so that, in the case of the current pandemic, government and healthcare leaders can negotiate effective responses to emerging needs, such as insufficient PPE, controlling infection rates, or implementing social distancing requirements and contact tracing.
I spoke with Dr. Andreas Tolk, who supports the COVID-19 Healthcare Coalition that MITRE sponsors. Characterizing modeling as a type of structured analytic, he told me that “we are in a deep uncertainty environment. Many [medical and epidemiological experts] do not know what theory is behind the different developments of coronavirus.” Models give those experts a credible foundation from which to work because the modelers consider theory, simulations, carefully observed situations, and constraints.
We might usefully view models as upstream tools that, in this case, limit the loss of life. By integrating observable factors and indicators, models help leaders and policymakers prevent problems from developing into greater crises. The governor of a state, say, might use modeling to make decisions such as mandating masks inside grocery stores and shutting down non-essential businesses, with the goal of limiting future infections and subsequent deaths. The governor’s response is upstream because it is attempting to limit the number of coronavirus cases by changing policy based on the models, instead of waiting for a downstream solution that would treat patients after they have contracted COVID-19.
Exploratory Analysis Techniques that Account for Human Behavior
How are scientists actually improving the accuracy of their models, given all the deep uncertainties of a virus as recent as COVID-19? At present, Dr. Tolk reported, exploratory analysis—the art of digging around in and cleaning data to prepare it for machine learning and theory-based algorithms—gives the scientists reliable information before they run their simulations. Consider an analogy: If the data used for the model is water, then exploratory analysis is the filter that removes harmful particles from the water.
Let’s examine what needs to be filtered when we’re talking about the greatest medical challenge in a century. This list isn’t meant to be exhaustive, but the subset of questions led Dr. Tolk to connect previous models of refugee populations to current models of COVID-19.
- How long will people obey stay-at-home orders?
- How often will people actually wear masks outdoors?
- Will all hospitals remain open and be able to pay staff when elective surgeries—their greatest source of revenue—are prohibited by law?
- Will deaths due to other conditions, such as heart disease, increase because people are afraid to seek medical help?
Because these questions all focus on human behavior, Dr. Tolk’s team realized that experts in the social sciences could help them create more accurate models. He observed that the “humanities and social sciences were driving what could be done [to better integrate refugees].” Specifically, as researchers at American and Norwegian universities modeled artificial societies to help guide humanitarian policy decisions, they took human behaviors into account.
Similarly, when behavioral scientists began looking at likely behaviors connected to COVID-19, they correctly predicted that people were only going to obey stay at home orders and mask mandates for 3-4 weeks before openly revolting and protesting mandates at state capitols. Thus armed, Dr. Tolk’s team of computer scientists then built data about those revolts into their models to create more accurate forecasts.
The salient words here are “more accurate.” Knowledge of human behavior is necessary, but AI/ML provides inferences that theories about human behavior cannot fill in by themselves. Dr. Tolk elaborated: “If we have a theory of how things work together, we can program a simulation that simulates the development based on this theory. If we don’t have a theory, we can still collect lots of data and use AI/ML to forecast based on learned trends. We often use hybrid approaches that employ simulation and AI/ML to combine the best of both worlds.”
For example, curve fitting, which creates a mathematical function based on data and simulations, is part of a hybrid approach. When curves related to the pandemic are combined with the results of millions of simulations based on limited medical and social theory, the resulting hybrid model can forecast PPE needs, policy-dependent death tolls, and other crucial medical and social factors.
Natural Language Processing Builds Efficiency into Research
A particular beneficiary of this AI forecasting approach is the MITRE-sponsored Healthcare Coalition, which comprises over a thousand private companies, all of which have one objective: defeating the coronavirus. In addition to benefitting from Dr. Tolk’s modeling efforts, the coalition has deployed natural language processing (NLP) to extract knowledge from enormous numbers of scholarly articles that document what works and what doesn’t.
Shortly after the coronavirus spread to America, the White House released the CORD-19 dataset, an open source collection of more than 57,000 scholarly articles and journals on COVID-19 and related medical knowledge. To understand how the burdensome task of sifting through tens of thousands of articles might be made easier, I spoke with Dr. Abigail Gertner and learned about MITRE’s CORD-19 Document Explorer and CORD-19 Topic Browser. The Document Explorer allows researchers to search and filter documents by topic, journal, or text-based query. Using an NLP technique known as word embedding, the Document Explorer helps researchers identify related terms (e.g., coronavirus and COVID-19) in order to modify and expand their searches. The Topic Browser, on the other hand, shows groupings and trends that allow researchers to see which topics are emerging as most important in the published research literature so that they can explore those articles. By handling a mechanical task efficiently, the Document Explorer saves thousands of hours and enables the researchers to do what humans do best: address the creative parts of the problems.
This article only scratches the surface of AI/ML’s role in combatting COVID-19. When I asked him about the bigger picture, Mr. Crossler responded that MITRE’s role in the national response to the pandemic is to give lawmakers and companies unbiased counsel and tools that can help with lifesaving decision-making. AI plays a crucial role in preventing the spread of the coronavirus, from using NLP to streamline life-saving research, to enacting model-driven social policies. The upstream potential of AI/ML is endless, and the stakes during this pandemic are life and death.
Omkar Ratnaparkhi worked as a Summer Student Investigator in the Emerging Technologies Department at MITRE. He engaged in public-interest reporting on leading-edge problem-solving approaches and technologies. A rising sophomore at Fordham University, he is majoring in International Political Economy, and plans to attend law school.
© 2020 The MITRE Corporation. All rights reserved. Approved for public release. Distribution unlimited. Case number 20-2140
MITRE’s mission-driven teams are dedicated to solving problems for a safer world. Through our public-private partnerships and federally funded R&D centers, we work across government and in partnership with industry to tackle challenges to the safety, stability, and well-being of our nation. Learn more about MITRE.
See also:
Working Upstream to Enrich Veteran Wellness and Prevent Suicide
Improve Your Resiliency Without Wearing Camouflage
Getting Students Excited About STEM (and MITRE), with Willie Hill
Going Virtual Can’t Hold Back MITRE’s 2020 Interns
Interview with Jay Crossler on Why Passion is the Key to Success
Creating an AI-Savvy Workforce for a Strong Future
Preparing for the Future by Knowing How to Take a Punch
The Emerging Technology Student Program’s New Frontier
Many Heads Are Always Better Than One
Changing Organizations Using the Power of Localism
Mistakes and Transcendent Paradoxes: Dr. Peter Senge Talks on Cultivating Learning Organizations