Applications in Data Science: Relevance and Anomalies
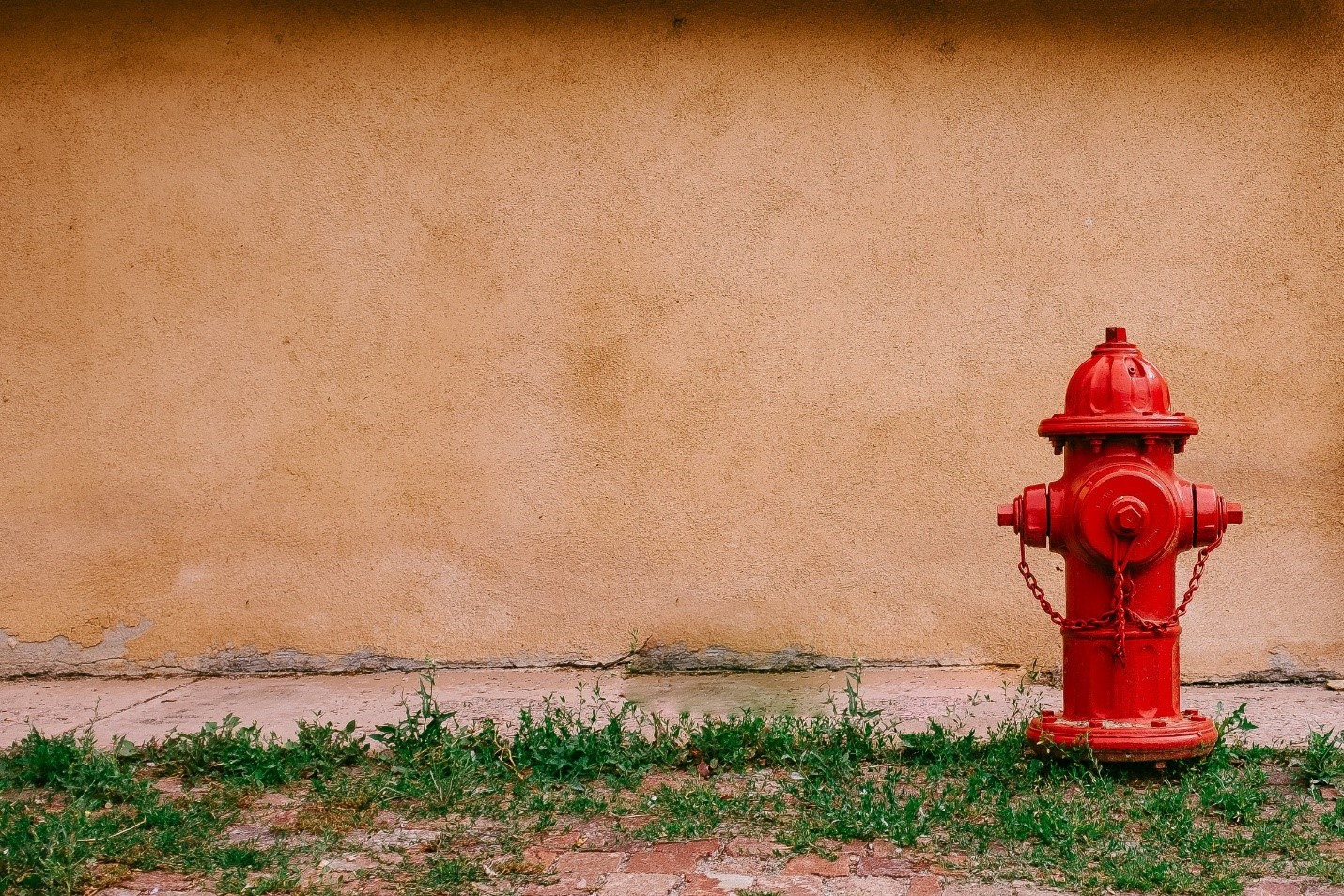
There’s thinking about, talking about, and doing, and they all have a time and place in any domain. With data science, though, doers rule. A big bucket of ostensibly random stuff in the hands of a skilled practitioner becomes the stuff of art. Yup, even data about a fire hydrant.—Editor
Photo by Unsplash
Author: Amanda Andrei
“What do we have?”
Simple and powerful, the first biggest data science question from Mike Shea, an engineer from the Global Operations Division, goes beyond data science. He elaborates: “What do we really have in this giant pile of data? And how do we even look at the other data to determine what’s there?”
And his second biggest question, “What don’t we have?” leads to more questions: “What data are relevant to what we’re trying to accomplish, that don’t exist, that we haven’t looked into? How do we identify that gap, not just with human beings identifying it, but statistically? How do we know what’s relevant?”
While Shea’s questions may seem overwhelming in the abstract, they are actually quite helpful when applied to specific problems, such as the document sorting discussed in Data Science Practitioners. Simply by identifying the types of documents in the data stream, he and his team were able to tell the sponsors which ones were relevant or not, thereby saving sponsors the trouble of collecting data they didn’t need. Or if you’re able to see what data isn’t there, you may be able to detect another pattern emerging. “There are random patterns you should witness and if those disappear, then something’s weird,” explains Shea. “It’s not following the normal distribution.” Patterns like this could point to social phenomena such as fraud or a physical issue such as a medical problem.
This presence of “something weird” – an event or observation that deviates from the pattern – could mean that there is an anomaly. And in data science, anomaly detection is crucial, needing both humans and machines to interpret the data and patterns. These interpretations may have huge impacts on social behavior or policy.
For instance, one of Shea’s favorite examples of simple data science – or, if you will, using simple but powerful techniques to look at huge amounts of data – is the problem of the New York City fire hydrants that unfairly netted $55,000 each year due to citizens parking in a space they did not realize was illegal. When a computer scientist blogged about how he had used NYC Open Data to discover the two outlier fire hydrants, the NYC government responded by repainting the lines on the road to clarify where to park. Citizens started saving thousands of dollars. It took a large amount of data to recognize this, as well as a human analyst to detect it.
“Algorithms tend to chase the middle of a distribution,” says Shea, meaning that computers are often trained to look towards the average of the majority. “If we get to a point where algorithms are determining more of what we see and read and are talking about—if we’re constantly aiming towards the average, we’ll miss those high-performing, highly impactful outliers, which will drive us to a better future.”
One of the ways to adjust for this is to have the right balance of human and computer aid. Shea gives the example of a computer-aided human, such as a weather forecaster using computer models to determine a hurricane’s impact, an example published in FiveThirtyEight founder and statistician Nate Silver’s book The Signal and the Noise (which Shea contends is the best data science book he’s read in the past few years). And on the other hand, there’s also the option of a human-aided computer, such as an online advertisement system that uses human input to determine customized ads for each user. Ultimately, Shea says it’s important to ask, “How do we carefully use the algorithms to augment people’s decision-making?” – a question data science teams should ask themselves as they go forward in designing and analyzing systems.
Did you enjoy this series on data science practitioners and applications in data science? Want to hear more stories? Let us know!
Amanda Andrei is a computational social scientist in the Department of Cognitive Sciences and Artificial Intelligence. She specializes in social media analysis, designing innovative spaces, and writing articles on cool subjects.
See also:
Technical Challenges in Data Science
Defining, Applying, and Coordinating Data Science at MITRE
Rising to the Challenge: Countering Unauthorized Unmanned Aircraft Systems
Mistakes and Transcendent Paradoxes: Dr. Peter Senge Talks on Cultivating Learning Organizations
MITRE Hackathon Examines Impact of Emerging Mobile Technologies
Digital Notebooks for Emergency First Responders
Managing Knowledge Through Systems Visioneering
© 2018 The MITRE Corporation. All rights reserved. Approved for public release. Distribution unlimited. Case number 18-0464
The MITRE Corporation is a not-for-profit organization that operates research and development centers sponsored by the federal government. Learn more about MITRE.