Interview with Dr. Philip Barry on blending AI and education
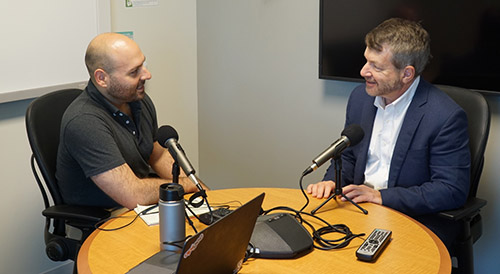
Cameron Boozarjomehri (left) interviewing Dr. Philip Barry (right). Photo: Lee Brown
Interviewer: Cameron Boozarjomehri
Welcome to the latest installment of the Knowledge-Driven Podcast. In this series, Software Systems Engineer Cameron Boozarjomehri interviews technical leaders at MITRE who have made knowledge sharing and collaboration an integral part of their practice.
Dr. Philip Barry is the Technical Director for Modeling, Simulation, Experiments, & Analysis here at MITRE. When he’s not leading simulations work, he is teaching Risk Management at George Mason. Ever focused on bringing new tools and methodologies into the classroom, Dr. Barry partnered with George Mason and Joe Garner and Ali Zaidi from MITRE’s Generation AI Nexus (Gen AI) team, to create a first-of-its-kind lesson blending risk management with artificial intelligence (AI). In this interview, we discuss Dr. Barry’s journey, not only in building the lesson, but also on expanding this effort to the larger George Mason Systems Engineering and Operations Research Program. Both are steps he sees as critical to training the technical leaders of the future.
Click below to listen to podcast:
Cameron: | 00:15 | [Intro music 00:00:00] Hello everyone and welcome to MITRE’s Knowledge-Driven Podcast. I’m your host, Cameron Boozarjomehri, and today I’m joined by a very special guest, MITRE’s Technical Director for Modeling, Simulation, Experimentation, and Analytics, Dr. Phil Barry. Hello, Phil. |
Philip: | 00:30 | Hi, how are you? |
Cameron: | 00:31 | Good. So how is work…are you enjoying your MITRE time? |
Philip: | 00:36 | MITRE is great. I’m a long-time MITRE guy, so we’re doing some exciting things. This is a relatively new job for me, and modeling simulation, experimentation, and analytics is going to change your life, Cameron. |
Cameron: | 00:47 | I believe it. And I think a good way to start is, as always, we like to get a little background on who we’re talking to. What was your… journey at MITRE coming into this position or what … brought you into this area of expertise? |
Philip: | 01:00 | So I came to MITRE a long, long time ago, over 20 years ago, as a software engineer, and back then I could actually code. Since then, I’ve been introduced to Modeling and Sim and worked in a government office for a number of years. Got away from it, and then recently this last year, there was an opportunity to do a job swap rotation, if you will, with the guy that was in the position, and I took it, and |
Cameron: | 01:33 | I appreciate the humbleness. Yeah, it’s well deserved because I’ve heard nothing but good things coming out of the simulation work. SIMEX is part of your department, right? |
Philip: | 01:40 | It is indeed, yes. We support SIMEXs. |
Cameron: | 01:42 | Simulation experimentation— |
Philip: | 01:44 | Right. |
Cameron: | 01:44 | Where we try to take on all sorts of different tasks for our sponsors and make sure that when they are faced with those tasks in the real world, it’s as close to the real thing as possible. So, I was excited to have you on because recently I had the opportunity to interview a colleague of yours, Ali Zaidi, and he was telling us about his work with Generation AI Nexus and you being his primary collaborator. I thought it’d be really fun to hear the other side of what it was like creating a curriculum around bringing artificial intelligence to … an actual academic setting. |
Philip: | 02:15 | So, as Ali told you, one of my side jobs is working at George Mason as an adjunct professor in Systems Engineering and Operations Research. And I started to talking to Ali and Michael Balazs, who runs the Gen AI effort down here at MITRE. And we started to think about where we could put AI, not as a centerpiece like in a computer science course, but actually as maybe partly embedded in the curriculum. So I went ahead and said, yeah, I’d like to do it. And then I had absolutely no idea what we were going to do. And so we started the course, and we start to think about risk management. |
Philip: | 02:55 | Now I’m gonna get a little preachy. So just work with me on this. Risk, as you probably know, is basically a couple of three things. One of which is an event or an action. The second, which is an outcome. And the third of which is a probability that’s going to occur. Now, quite often in the system engineering side, the way we estimate risk is on Kentucky windage. Have you heard of Kentucky Windage? |
Cameron: | 03:17 | I’ve never heard of that expression. |
Philip: | 03:18 | It’s when you put your thumb up in the air and go: “That’s sort of, kind of windy today”. In other words, we’re guessing. |
Cameron: | 03:23 | Hmm. |
Philip: | 03:24 | We do something called heuristics, where we use expert opinion but it’s not data-driven. So one of the challenges—and really credit to Ali and his collaborator Joe Garner—was, I said, “Is there something we can do here in the risk area to maybe bring in data analytics and machine learning to switch the way we’re doing risk assessment?” Now, just by way of background, one of the things that we do in this course at George Mason is I give them a project in the beginning of the semester and every week, we have a lecture and then they have an assignment that is based on that lecture. One of which happens to be risk. So the project was to come up with an autonomous bus system for George Mason to go all the way from the Fairfax Campus to the Manassas Campus. |
Cameron: | 04:11 | That is not a simple task. |
Philip: | 04:12 | That is not a simple task. And if you think about it, there are risks aplenty, but where would you come up with the estimate for it? How likely is that risk going to happen? Where’d you come up with the impact for that? |
Cameron: | 04:25 | Especially when it’s something that doesn’t exist. |
Philip: | 04:28 | Exactly. Exactly. So that’s where we said, let’s see if we can look around, find a bunch of data on where things have failed and maybe do some reasoning by analogy and saying, this project is sorta like that. So I pitched my boss over at George Mason, and we did that, I guess, in December, January of this year. And he said, sure. We didn’t actually pitch them on the idea because at that time we didn’t know what we’re doing. And then over the next several weeks, Ali and Joe not only came up with the curriculum but came up with a way to do data-driven risk estimation. And that’s what we gave the students to change the way they think about risk. |
Cameron: | 05:07 | And that’s actually the way you set this up, it’s something I really want to kind of jump into because you mentioned that you want it to be integrated with the curriculum and not just a center piece of the curriculum. You’re saying you don’t want to just spend like a module or two focused on AI. You’re trying to say that this thing, this technology is something that is going to become part of how, at the very least, our industry does what it does moving forward, and we need to make it clear that this isn’t just something that you might come across. This is going to become part of your everyday experience doing this work in risk management. |
Philip: | 05:40 | And I think that, I think it has the opportunity. I’ll give you an example. I’m old enough to remember when computers first hit the streets. My daughter, who is now 14, has never lived in a world where she didn’t have the answer to every single question in her hand. That’s the way she approaches the world. I’m a digital immigrant. She’s a digital native, which is very different. That’s what we’re trying to do with Gen AI—pushing this idea of machine learning and data analytics way down into even, into grade school, to change the way people think. So we’re starting at the college level. We’re starting, and I teach a graduate course, but the idea is that this is embedded in the way people think. So it would be embedded in risk management; it might be embedded in scheduling; it might be embedded in other things just within the project domain. And, of course, within systems engineering writ large, there’s many opportunities for machine learning, AI, and that sort of thing. |
Cameron: | 06:36 | I think this actually dives into very important part, this distinction between digital natives and digital immigrants because a lot of the people that I think are running industries now, they came about, their careers came about as CTOs and whatnot. When technology was itself kind of coming to be what it is, what we understand today. It wasn’t always computers and the software and the tools we have now. That was something that over time has been built and learned. But there are a lot of people born right now, and I not sure if I’m young enough to be included with them. |
Philip: | 07:10 | You might be right on the edge Cameron, just teetering over. Yeah, there you go. |
Cameron: | 07:14 | But they’ve been raised in a world where they have touch screens, they have this way of accessing information. That real benefit is understanding how to parcel that information, how to stitch it together to create new ideas or identify overlooked patterns and old ones. |
Philip: | 07:29 | I’ll give you an example. I do a little bit of work with the University of Virginia (National Champions this year I might point out), where I went to school and when I was in engineering school back in the 80s, yes, we did go to engineering school in the 1980s. We were in large part math monkeys. I’d go to fluid mechanics courses, and one of the things they would say is, here’s a bunch of equations and I’d go in the library and pound my head on the desk or a number of hours until I could finally understand it. No one does that anymore. A math lab, why would you do that? So you can do the engineering. It’s a shift in the way we do things. |
Philip: | 08:03 | I think those of us that have been working with the Gen AI see this as a shift in the way we can actually deliver education, not just for engineering businesses. Well, maybe even some of the liberal arts. We start to think about, here’s an application on how we can start to think: make it digitally driven, data driven rather, and also you can almost think about having explorative capability, build a model, explore how this works. Interpreting. |
Cameron: | 08:30 | And I think this does walk us into kind of an area we keep coming back to as we talk about not just Gen AI, but AI as a whole, which is there’s an intimidation factor that comes with all of this. It’s one thing to understand that AI is going to help us do better, be better, but it’s another thing to appreciate that people are kind of nervous about it. They don’t necessarily understand this technology. A lot of people when they learn about AI, they’re learning at the nuts and bolts level of, this is how you implement this algorithm and not at the, as a person who’s being delivered data, how do I slice it and parse it and put it back together using this new tool I have. And I think this is a critical thing to tie back into your curriculum because of how you’ve approached it with especially this bus example, saying that this is, this is a way that these tools are just there. How do these tools benefit my students or benefit their goals? |
Philip: | 09:25 | I think that’s a great point. And one of the things when I took AI, when I was in graduate school, they’d give us a bunch of C++ code and we would have to go ahead— |
Cameron: | 09:34 | Oh no. |
Philip: | 09:35 | Yeah, I had to learn C++ on my own by the way, and we would do things like genetic algorithms and classifier systems, and such like that. For the class, the project management course that we took, Ali created a Python notebook and all of that code, the necessity to do the code was by and large hidden. I’ll give you another example. You probably have an iPhone or something like that, and you can use Siri and Siri is not intimidating. Everybody uses Siri or Alexa or whatever the Google version of that is, and one of the things is it’s not intimidating if it’s not a huge new skill you have to learn. |
Philip: | 10:16 | And if you look at what Ali did, it looks sort of like an Excel spreadsheet. People are very comfortable with that. So as we start to bring this into the curriculum, you’re exactly right. If we say we’re going to make you learn AI, so you have to learn a TensorFlow package. Oh my gosh, nobody wants to do that. Particularly people that are not computer scientists. But if we— |
Cameron: | 10:36 | The word TensorFlow just makes me cringe right there. |
Philip: | 10:38 | Yeah, right. It’s really cool by the way. |
Cameron: | 10:40 | It is. |
Philip: | 10:41 | But if you say, you know what, we’re going to give you a Python page or a Jupiter notebook rather, which is what Ali did, and here are some things you can do with the data and all you have to do is change these variables and look at how it displays. Well, there’s some data analytics there and you’re not really learning the data analytic machinations under it, you’re using it as a tool. |
Philip: | 11:03 | Give you another simple example. Do you really understand how your car works? |
Cameron: | 11:08 | I know that gas goes in and tires spin. |
Philip: | 11:10 | Right, gas goes in and tires spin. Back in the day, if you, long, long time, even before when I had a car, people understood the engine. There is so much electronics in that, very few people really understand what happens, but are you intimidated with your car? No, of course not. You drive to work every day, you go see your friends, et cetera. Make this a tool just like a spreadsheet so that people get comfortable, but you’re exactly right. You see, you say AI, people think Terminator and things like that and it’s going to take over. To be fair, the world’s gonna change. Absolutely. |
Cameron: | 11:44 | And this, I feel like this is an important shift because the goal is not to say AI is a replacement for people. AI is kind of an add-on, a collaborative tool. I think a lot of people, when they think about artificial intelligence, they think, this algorithm is going to do my job better than I ever could, and now I’m done. When, in reality, it’s you are still the person pushing whatever that effort is. You’re expected to be the person making laws, you’re expected to be the person planning cities, whatever. And the machines aren’t just going to do that for you, but they are going to be able to show you when people play in cities, the way you’re about to, this is what typically happened and is that the outcome you wanted? And I think that this goes back to your course because you can do that at different scales. |
Cameron: | 12:32 | There’s like, we might not need everyone to become city planners, but you were trying to integrate, I guess I should step back because it’s one thing that as I understand this was a graduate course, and when I was in grad school, I just kind of had to do whatever. But I feel like when people, when you put this in all levels of academia, this kind of work, depending on how excited people get about it or how easy it is to kind of latch onto the bigger ideas around it, really impact whether people are naturally pulled towards that work. And I’m, I guess what I’m saying is what, I’m curious to see what kind of challenges you’re facing in terms of making this not just a grad-level course, but a people-going-through-academia-at-all-levels-of-systems engineering course. |
Philip: | 13:16 | Well, I’d be reticent to say I can answer that at large. I’ll certainly give you my opinion on it. One of the things that came out of this that was interesting was when we looked at the feedback from the students, they said, “Why are you doing this? Why are you putting this in the curriculum?” We’re taking a course in project management. My guess is that particularly as you begin to go away from the STEM-related courses, and you start to get maybe into the more liberal arts…. I was talking to Jay Crossler, the Chief Engineer here, and he was talking about how they’re doing this in an art history class, machine learning for art history, which I found fascinating. |
Philip: | 13:54 | Now that’s certainly a guild, if you will, an expertise that hasn’t used a whole lot of machine learning and AI. I think you’re going to get a little bit more resistance from the digital immigrants. Now, take my daughter for example, she’s 14, she taught herself how to code. She had three friends and they were doing collaborative coding and they were collaboratively working on something and they’re located all across northern Virginia. |
Cameron: | 14:22 | That is absolutely amazing. it’s great to hear. I think we especially live in an age, as you point out, we have so much data at our fingertips. If I really want to learn something, I just go learn it. I don’t have to wait for permission or go to school to do it. |
Philip: | 14:33 | So that’s where I’m thinking I’m very optimistic, which is I don’t have to convince people of that generation. One other thing, by the way, and this is the keep-you-awake-at-night fact, China is doing this at scale, so it’s almost a national security issue. China is pushing AI and computer science down to grade school, at scale, and they have three times more many people than we do, actually four times more. So it’s a national security issue, something that we should be doing, not just because it’s cool, which it is, but it’s actually great for the nation to have people to do this. Imagine if you will, as opposed to having just computer scientists and artificial intelligence specialists working on it, you had artists thinking about that. And that’s really when you start to think about how they design things like the iPhone. It wasn’t just a bunch of people that thought linearly. It was a diverse viewpoint that Frans Johannson entered at the intersection-type discussion. |
Cameron: | 15:31 | And this actually goes, I feel like this goes to a broader discussion about diversity of ideas. It’s one thing to have a bunch of people who know how to build an application or a system, or how to build an application for your system. It’s another thing to give people who have to use those tools who have to interact with whatever systems you’re building, the language and knowledge to really speak to what’s working, what’s not, what they know that they can share with you so that you can both be on the same page quicker. |
Philip: | 15:56 | So I’m going to give a shameless plug for simulations right now. |
Cameron: | 15:59 | Go for it. |
Philip: | 16:01 | If you’ve heard of the term counterfactuals, the “what if”. What if we change this, how would it look? That’s what simulations can do. And that’s also what some of these AI systems can do. If you have a recommender system, you know, do you go to restaurants in a city that you’re not familiar with without looking at Yelp? I don’t. There’s a recommender system now. It’s a crowdsource. Now suppose there was an algorithm, it said Cameron looking at the restaurants you’ve gone to over the last year, we recommend, where do we have that? Oh, Amazon. |
Cameron: | 16:30 | Yes. |
Philip: | 16:30 | Right. |
Cameron: | 16:31 | I gotta be honest. Sometimes I find those algorithms very annoying because I buy a watch and then it spends the rest of the month trying to sell me another one. |
Philip: | 16:38 | Another watch. |
Cameron: | 16:38 | [crosstalk] That’s like why I already have a watch. Thank you though. |
Philip: | 16:40 | But think about this. Think about if you could do this deliberately. Think about if you had somebody in an algorithm that you could actually ask, and that’s sort of where we’re going now. Is that a scary thing? Well, it might be a little eerie. I was on Home Depot trying to get some information. I realized I was talking to a chatbot. |
Cameron: | 16:58 | Okay. That and I feel like I’d be a little turned off, I guess that would be the term if I found out I was talking to a chatbot and not people. [crosstalk] |
Philip: | 17:05 | As I realized that the “conversation,” and I use that term in quotes, was going nowhere, right. But I guess the point of the matter is, going back to the Gen AI, two things are happening, one of which is we have a generation coming up that have always had this. The second thing is that the technology is improving by leaps and bounds, so by the time my daughter enters the workforce, think about that. Think about where technology was 12 years ago compared to where it is now. It’s funny, if you ever watch a movie and it was a movie from the early 2000s you look at their phones. |
Cameron: | 17:33 | Yeah, they’re— |
Philip: | 17:34 | Flip phones. |
Cameron: | 17:34 | Or bricks. |
Philip: | 17:35 | Or bricks, right, so now this Gen AI thing, the idea is to get people comfortable with this and not everybody’s going to be writing algorithms, but maybe everybody’s comfortable using the capabilities and the tools and thinking about the possibility space. That’s what I’m excited about. |
Cameron: | 17:52 | And I think an important distinction here is that I don’t think people are afraid of AI so much as intimidated by it. It’s people who grow up in a world only knowing technology and AI. This feels like something that’s natural or something that they’re eventually just going to be interacting with anyway. But people who spent all their time growing up in a world where they use typewriters, they used land lines, and suddenly you have this thing in your pocket that gives you all this access. |
Cameron: | 18:21 | Yes, on the one hand, it’s really easy to see all the benefits. On the other hand, it can be intimidating when you’re finding out that this is the future of how you are going to work and it’s not great to just shove people and say, this is your life now, accept it. That’s what makes this program, I feel like this is why we’re always talking about it. This constant effort by MITRE to show that like we are here to work with you, to teach you. You’re not just getting thrown in the water and expected to sink or swim. We’re giving you, not a lifeline, we’re giving you a raft and teaching you how to use it. |
Philip: | 18:54 | Yeah. I think the, one of the differences is it goes back to this idea of counterfactuals. We start thinking about what if, what if I could do this? What if I could do this? Then it’s a little less intimidating. So when we start thinking about you know, more in the AI field, our goal I think in Gen AI is to integrate this into the curriculum, certainly in the stem fields but also in the other things where this is a possibility, this is a tool, this is your paintbrush if you will, that you can use or not. It’s not, “you are now a systems engineer, you are now going to use this, you are now an ops research analyst. You’re now going to use this data analytics”. |
Philip: | 19:30 | And then as the technology matures and people are generally curious, I mean some people are going to go ahead and be Eeyores and not want to jump on board, but I think it will come up, come along and then again not to keep pounding the table on this and you told me not to pound the table. When you start thinking about digital natives, certainly that generation behind you, that’s all they’ve ever known. |
Cameron: | 19:50 | I think one last important thing to get at is this divide of digital natives and digital immigrants in terms of, you had to get buy in from someone to even get this to be a part of your curriculum. I’m curious if you could speak to what those conversations are like about showing people this is a thing that we’re doing to help us. |
Philip: | 20:07 | When I started talking to my boss over at George Mason, I said, you know, this is the where the field is moving, not just MITRE. And we start to think about the curriculum, where are opportunities both to enhance what we do for systems engineering as well as think about how our systems engineering approach needs to change to field systems that are AI intensive. And he said, sure, go ahead. And when we briefed him about a month ago, it turns out that it went out really well. Sort of a pilot and hopefully we’ll have the opportunity, you mentioned maybe talking to the curriculum committee. Just think about a broader application. |
Cameron: | 20:43 | And I think a good final point is if people are curious to learn more about Gen AI or specifically your work, bringing this into systems engineering and risk management. What kind of resources can they look for or reach out? |
Philip Barry: | 20:54 | Within MITRE, Michael Balazs is leading the Gen AI effort and he can certainly talk to that in the broader sense and you can certainly call, talk to me within MITRE about things that I’ve done specifically in risk management and trying to influence the systems engineering curriculum at George Mason. In the broader world, I believe, see me as outwardly facing. There’s also an effort I’m aware of through the Stevens Institute, a Systems Engineering Research Consortium, SIRC, is coming up with a research agenda for how to integrate AI and systems engineering, and that would probably be a good place to start. |
Philip: | 21:34 | Here’s my challenge to anybody that’s listening to this. Don’t watch. Jump in what’s going on. If you’re at MITRE, certainly learn about Gen AI. It doesn’t necessarily mean you have to participate. Start to think about, you know what? What is the possibility space. If I wanted to do something in my job, maybe my research interests, maybe even in my personal life, what would be really good? And it’s sort of fascinating for this as I’ve been here for a while. I just think about 10 years ago where things are and where things are now. This is happening and I have what I call the WTOP challenge. If you don’t believe me, next time you’re going into work, turn on WTOP here in Washington and if you don’t hear AI and machine learning five times before you get to work, I’ll buy you a cup of coffee. |
Cameron: | 22:22 | As the authentic Dr. Phillip Barry promise. |
Philip: | 22:26 | Exactly. |
Cameron: | 22:26 | Thank you so much for coming on and talking with us. I’d like to give a quick thank you to MITRE and the Knowledge-Driven Enterprise for making this show possible. And again, thank you so much for your incredible work building this curriculum, and I think we’re all excited to hear what happens next time, what those conversations for the future of AI inside systems engineering looks like. |
Philip: | 22:47 | Well, this is fun. I appreciate you having me on, and I’ll look forward to hearing what I said. |
Cameron: | 22:51 | Awesome. |
Philip: | 22:52 | Thanks. |
Cameron Boozarjomehri is a Software Engineer and a member of MITRE’s Privacy Capability. His passion is exploring the applications and implications of emerging technologies and finding new ways to make those technologies accessible to the public.
© 2019 The MITRE Corporation. All rights reserved. Approved for public release. Distribution unlimited. (Case number 19-2238)
MITRE’s mission-driven team is dedicated to solving problems for a safer world. Learn more about MITRE.
See also:
Interview with Ali Zaidi on Designing Lessons in Artificial Intelligence
Interview with Dan Ward, Rachel Gregorio, and Jessica Yu on MITRE’s Innovation Toolkit
Interview with Tammy Freeman on Redefining Innovation
Interview with Jesse Buonanno on Blockchain
Interview with Dr. Michael Balazs on Generation AI Nexus
Interview with Dr. Sanith Wijesinghe on Agile Connected Government
Is This a Wolf? Understanding Bias in Machine Learning
A Spin Around the Blockchain—Exploring Future Government Applications