Cultural Challenges in Data Science
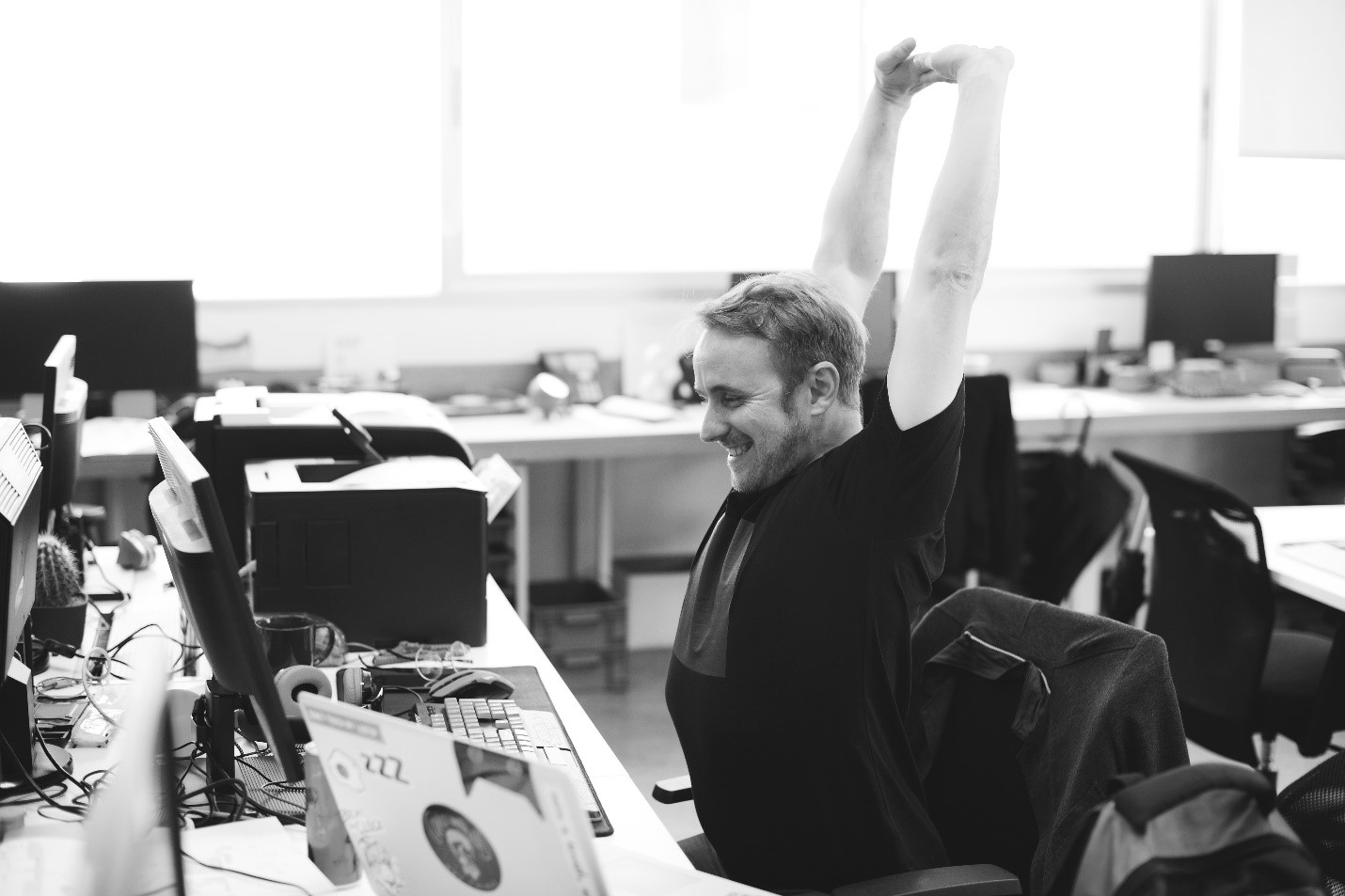
In her previous post, Technical Challenges in Data Science, Amanda Andrei discussed the need for technical vigilance with experts Dr. Elizabeth Hohman, statistician and group leader within MITRE’s Department of Data Analytics, and Dr. Eric Bloedorn, senior principal artificial intelligence engineer. Tools and models, however carefully managed, tell only part of the story, of course. Data scientists are people, and they and the tools they use reside within organizational cultures, which may require as much training as the data to hand.—Editor
Public domain photo courtesy of Unsplash
Author: Amanda L. Andrei
Cultural challenges encompass the ways that people work together when thinking about and using technical techniques to tackle data science issues.
Creating a solid data science team. As addressed in Defining, Applying, and Coordinating Data Science at MITRE, the key roles for a data science team are a domain subject matter expert, a hybrid data scientist and domain subject matter expert, and a modeling subject matter expert, all of whom communicate well with each other and are as tightly coordinated as a football team. Expanding upon this concept, Dr. Eric Bloedorn, senior principal artificial intelligence engineer, and Bernard McShea, a lead cyber security engineer, suggest that the team should accomplish the following:
- Define the scope, approach, and model maintenance for the most important challenges
- Establish sound features to build on
- Solve problems that have a wide impact
- Solve problems that will shape the domain landscape
Once the team has a few working models, the tasks can be passed on to another data scientist, ideally one newer to the field and in need of more experience, thereby training a new data scientist, saving sponsors more resources, and allowing the main team to address more challenges. Using this team approach—as opposed to attempting to find one individual who can “do everything”—provides rigorous evaluation and domain credibility to the problems at hand. And it nourishes a pipeline of up-and-coming data scientists who are as skilled as they are passionate.
Thinking beyond tools. Similar to the technical challenge of using appropriate training data, researchers should think carefully about using appropriate tools. “People say, I like this particular tool, I’ll use this, or, I know the domain, I’ll push it through a neural network and that’ll do it for me,” relates Bloedorn. “You’ll get some answer back, but what it means and how good it is, is not always clearly evident.” Answers could include spurious correlations, invalid inferences, and an approach that other scientists are unable to reproduce. Assembling a solid data science team may help the sponsor avoid some of these issues, but it is still important for team members to not default to their preferred tools or methods, and instead collaborate to find a reproducible, defensible answer to their questions.
Cross-training people long-term in data science. The MITRE Institute, the corporation’s center for education and development, offers many resources for learning data science techniques and tools. Most notably, the MITRE Institute offers Learning Paths, a set of courses centered around a relevant topic, such as Big Data for Analytics. MITRE staff and government participants receive an introduction to theory and hands-on lab experience with various tools and systems. A domain expert with a background in healthcare or aviation safety can enroll in the learning path and gain knowledge and vocabulary for the more technical details involved in data science.
However, to be an effective data scientist, participants need to work on more examples and be paired with more experienced data scientists to apply what they learned in a classroom to problems they encounter in the real world. “In the real world, you don’t get an assignment and say, Everything else is done, just do this assignment,” shares Bloedorn. “Instead someone says, Here’s a pile of data, my analysts are overwhelmed, help me. So you need to be able to form the question and figure the analytics to form that question.” Ultimately, a well-trained person or team will be able to do precisely that—form the right question to the problem and determine which analytics to use in understanding or solving the problem.
Amanda Andrei is a computational social scientist in the Department of Cognitive Sciences and Artificial Intelligence. She specializes in social media analysis, designing innovative spaces, and writing articles on cool subjects.
See also:
Technical Challenges in Data Science
Defining, Applying, and Coordinating Data Science at MITRE
Rising to the Challenge: Countering Unauthorized Unmanned Aircraft Systems
Mistakes and Transcendent Paradoxes: Dr. Peter Senge Talks on Cultivating Learning Organizations
MITRE Hackathon Examines Impact of Emerging Mobile Technologies
Digital Notebooks for Emergency First Responders
Managing Knowledge Through Systems Visioneering
© 2017 The MITRE Corporation. All rights reserved. Approved for public release. Distribution unlimited. Case number: 17-4606.
The MITRE Corporation is a not-for-profit organization that operates research and development centers sponsored by the federal government. Learn more about MITRE.
0 Comments